Project Team
- Taejoon Kim (KU)
- Chris Brinton (Purdue)
- Morteza Hashemi (KU)
- David Love (Purdue)
- James Krogmeier (Purdue)
Project Vision
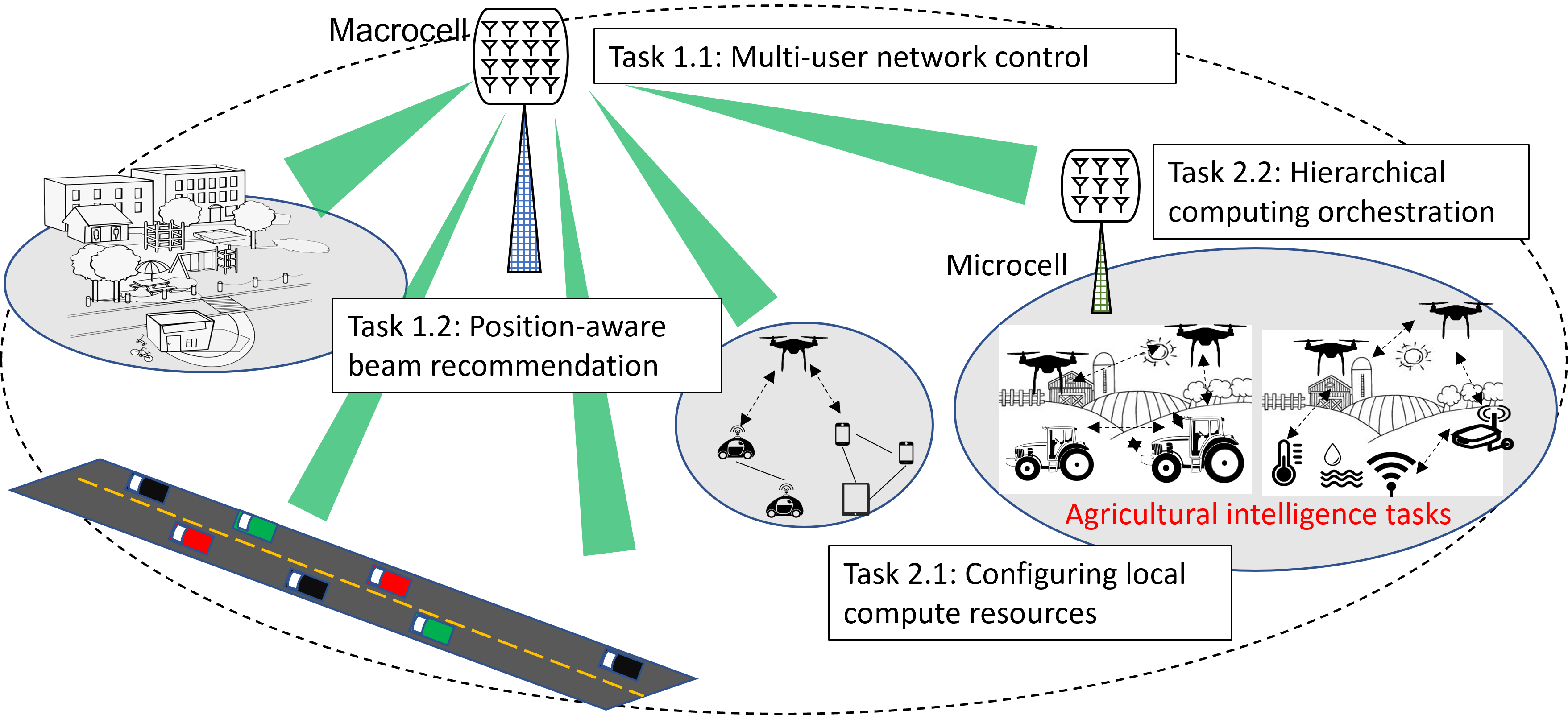
Remote consumer “islands” with non-existing infrastructure make rural areas less attractive for investment in network connectivity. 5G’s support for the integration of vertical industries coupled with the increasing demands for intelligent and analytics-driven use cases threaten to further magnify the socioeconomic consequences of broadband access inequalities. Technologies that have been proposed for rural connectivity over the years have ignored fundamental challenges arising from non-uniformity in the availability of, and demand for, communication and computation resources in rural settings. This non-uniformity manifests in several dimensions: (i) Heterogeneous devices and use cases, ranging from Internet of Things (IoT) to modern farming equipment, and from remote student learning to command-and-control of autonomous field robots; (ii) Temporal diversity, ranging from near-real-time agricultural machinery requirements to coarse-granular seasonal changes on top of standard diurnal patterns; (iii) Spatial diversity due to high skewness in user density across different areas. The research in this project will establish a core set of methodologies for addressing the non-uniformity requirements of rural networking. This will be achieved by integrating the design of massive multiple-input multiple-output (MIMO) connectivity with the orchestration of hierarchical data processing architectures across existing rural infrastructure. Additionally, extensive on-site evaluations of the methodologies will be conducted through the GOALI industrial partner, Blue Danube Systems (BDS) Inc., leveraging their macrocell massive MIMO platform and wireless device equipment.
Our overarching goal is to develop a core set of methodologies for addressing the fundamental communication and computation non-uniformity requirements of rural networking. We will achieve this by integrating network connectivity with the orchestration of hierarchical data processing architectures across heterogeneous device deployments, adapting to the unique spatial and temporal demand variations of different rural use cases. To achieve this, the following major goals are considered:

Goal 1–Agile Communications under Non-uniform Rural Settings: This thrust will address non-uniform multi-user management and communication resource allocation. We will develop novel network control algorithms with scheduling policies that are customized for heterogeneous rural connectivity needs. We will further investigate algorithms for fast link setup to reduce scheduling overhead, through position-aided channel representation techniques.
Goal 2–Computation Orchestration under Rural Non-uniformity: This thrust will focus on establishing rural task processing architectures to address computation non-uniformity. We will first investigate techniques for localizing data processing among clusters of rural devices aiming to execute rapid collaborative tasks, via joint optimization of D2D communication, device computation, and task utility metrics. We will then consider orchestrating data processing and intelligence synchronization steps along the rural network hierarchy to adapt based on spatial and temporal variations in task demand.
Goal 3–Evaluation: Proof-of-Concept Implementation and Testing: This thrust will evaluate the efficacy of the methodologies developed in Thrusts I and II based on simulations and proof-of-concept testbed implementation.
In addition to the Intellectual Merits, this project will have significant societal, economic, and research impacts. The rural networking solutions enabled by this project will enhance the ability of these communities to progress in the educational, societal, and business sectors. The proof-of-concept validation and integration with the commercial testbed from the GOALI partner will have a significant impact on the research community by providing unique wireless evaluation data from licensed bands in rural settings. The research in this project will be integrated with undergraduate- and graduate-level course offerings. Dissemination of the research results through the communications, computing, and agricultural areas will spur further development at the intersection of these communities.
Intellectural Merit
To address Goal 1, we divided our research efforts into the following tasks in Year 1 of the project:- As the first research task, we considered semantic and goal-oriented communications. Semantic communication is focused on optimizing the exchange of information by transmitting only the most relevant data required to convey the intended message to the receiver and achieve the desired communication goal. In this research task, we proposed a flexible framework for implementing semantic-aware and goal-oriented communication. To achieve this, we first define the baseline problem as a set of mathematical problems that can be optimized to improve the efficiency and effectiveness of the communication system. To make our development more concrete and grounded, we implement our proposed optimization problems for a specific semantic communication system with the goal of object detection at the receiver. This is relevant to several rural applications and use cases, such as object detection by UAVs and/or agricultural robots. We also introduce a practical framework to implement semantic communication using the auto-encoder architectures. Our numerical results validate the proposed design framework to achieve high accuracy in a goal-oriented communication system while reducing the amount of data transfers.
- In the second task, we embarked on formulating refined sensor selection strategies for 3D wireless positioning, primarily emphasizing optimizations using time of arrival and received signal strength measurements. These devised strategies are envisioned to be pivotal for the creation of innovative network control algorithms, tailored for the intricacies of non-uniform multi-user management in varied rural settings. Our focus on dynamic sensor selection and the pursuit of enhanced positioning accuracy, represented by the CRLB metric, underscores our commitment to harnessing position-aided channel representation techniques. Such initiatives are essential in facilitating swift link establishments, thereby minimizing scheduling overheads, a crucial move to optimize communication resource allocation in rural areas. Beyond the technological strides, this approach holds significant relevance for an array of rural applications and scenarios, ensuring connectivity is molded to their unique prerequisites.
- In the first research task, we have considered personalized Federated learning to address computing non-uniformity in rural applications. In particular, selecting the most suitable federation cluster for users to join in a dynamic federated learning system remains a challenge. In this research task, we leverage multi-armed bandit (MAB) approaches to propose a dynamic Upper Confidence Bound (dUCB) algorithm to achieve efficient, personalized federated learning under non-uniformity. Our algorithm adapts to the dynamic nature of rural learning applications by considering changes in data distributions, user behavior, and environmental factors. We extend the traditional UCB algorithm by incorporating a reward function that balances model performance and helps the new user find the optimal cluster for federation. Our proposed dUCB algorithm ensures that new users can effectively find the best cluster for their data distribution by balancing exploration and exploitation in dynamic federated learning scenarios.
- In the second research task, we extended our investigation into edge computing networks and considered the problem of joint communication-computation, and computation offloading in multi-channel systems with non-uniform properties. We developed online learning solutions using reinforcement learning techniques so that the mobile user can dynamically adjust its communication-computation policy for completing its computation tasks.
- The third research task centers on establishing rural task processing architectures to tackle computational non-uniformity, we ventured beyond conventional methods. Recognizing the importance of localized data processing among clusters of rural devices, we drew parallels from our work on maMIMO networks. In that research, we employed deep neural networks (DNNs) to ensure efficient power allocation, addressing the challenges of interference and computational overhead. Yet, the vulnerabilities we identified, particularly adversarial attacks on DNNs, provided a stark insight: the need for fortified, resilient computational systems is paramount. By successfully defending power allocation DNN models with denoising autoencoders (DAEs), we not only enhanced maMIMO systems but also laid the foundational groundwork for rural task processing. Using the knowledge gained, we now aspire to localize data processing and facilitate rapid collaborative tasks in rural networks. This involves a synchronized approach, integrating D2D communication, device computation, and task utility metrics. Furthermore, our endeavors will also focus on orchestrating data processing steps along the rural network hierarchy, ensuring adaptability in the face of spatial and temporal variations in task demand.
Broader Impacts Activities
The KU PIs have established a wireless summer camp at KU for high school students to teach them the fundamentals of signal processing, wireless communication, and hardware components. In this camp, the students become familiar with software-defined radios (SDR) and use Pluto SDR to conduct various wireless experiments. In summer 2023, 28 students enrolled in the wireless summer camp, which was held over two weeks (16 students in week 1 and 12 students in week 2).
Publications
- D. Q. Nguyen and T. Kim, “On the Stability of Approximate Message Passing with Independent Measurement Ensembles,” IEEE International Symposium on Information Theory (ISIT), June 2023.
- D. Q. Nguyen and T. Kim, “Joint Hybrid Delay-Phase Precoding Under True-Time Delay Constraints in Wideband Sub-THz Massive MIMO Systems,” submitted to IEEE Transactions on Communications, 2023.
- Oh, Myeung Suk, Seyyedali Hosseinalipour, Taejoon Kim, David J. Love, James V. Krogmeier, and Christopher G. Brinton. "Dynamic and Robust Sensor Selection Strategies for Wireless Positioning with TOA/RSS Measurement." IEEE Transactions on Vehicular Technology (2023).
- Kim, Junghoon, Seyyedali Hosseinalipour, Taejoon Kim, David J. Love, and Christopher G. Brinton. "Linear Coding for Gaussian Two-Way Channels." In 2022 58th Annual Allerton Conference on Communication, Control, and Computing (Allerton), pp. 1-8. IEEE, 2022.
- Ruzomberka, Eric, Homa Nikbakht, Christopher G. Brinton, David J. Love, and H. Vincent Poor. "Derandomizing Codes for the Binary Adversarial Wiretap Channel of Type II." arXiv preprint arXiv:2307.04222 (2023).
- Bliss, Matthew, Chih-Chun Wang, and David J. Love. "Optimal Single-Bit Relaying Strategies with Multi-Relay Diversity." IEEE Transactions on Information Theory (2023).
- Ogbe, Dennis, Chih-Chun Wang, and David J. Love. "On The Optimal Delay Growth Rate of Multi-hop Line Networks: Asymptotically Delay-Optimal Designs And The Corresponding Error Exponents." IEEE Transactions on Information Theory (2023).
- Ruzomberka, Eric, Yongkyu Jang, David J. Love, and H. Vincent Poor. "Adversarial Channels with O (1)-Bit Partial Feedback." arXiv preprint arXiv:2305.14541 (2023).
- J. Kim, T. Kim, D. J. Love, and C. G. Brinton, "Robust Non-Linear Feedback Coding via Power-Constrained Deep Learning", International Conference on Machine Learning (ICML), 2023.
- Sahay, Rajeev, Minjun Zhang, David J. Love, and Christopher G. Brinton. "Defending Adversarial Attacks on Deep Learning-Based Power Allocation in Massive MIMO Using Denoising Autoencoders." IEEE Transactions on Cognitive Communications and Networking (2023).
- M. Ghazikor, K. Roach, K. Cheung, M. Hashemi, “Exploring the Interplay of Interference and Queues in Unlicensed Spectrum Bands for UAV Networks”, Asilomar Conference on Signals, Systems, and Computers, 2023.
- B. Badnava, K. Roach, K. Cheung, M. Hashemi, N. B. Shroff, “Energy-Efficient Deadline-Aware Edge Computing: Bandit Learning with Partial Observations in Multi-Channel Systems”, Globecom 2023.
- S. Chintareddy, K. Roach, K. Cheung, M. Hashemi, “Collaborative Wideband Spectrum Sensing and Scheduling for Networked UAVs in UTM Systems”, Globecom 2023.
- A. Shojaeighadikolaei, M. Hashemi, “An Efficient Distributed Multi-Agent Reinforcement Learning for EV Charging Network Control”, Allerton 2023
Graduate Research Assistants & Collaborators
Graduate Research Assistants:
- Wai Ming Chan
- Masoud Ghazikor
- Ahmed Mohamed
- Fatemeh Zahra
- Satyavrat Wagle
Collaborators:
- H. V. Poor (Princeton, USA)
- E. Ruzomberka (Princeton, USA)
- J. Choi (KAIST, Korea)
- S. Hosseinalipour (University of Buffalo, USA)
- Yongkyu Jang (Purdue, USA)